Exploiting large ensembles for a better yet simpler climate model evaluation
SMILE experiments consist of many simulations of a single climate model under the same time-evolving external forcings, but starting from different initial conditions. The authors used them to bridge differences between observations and simulations. Observations reflect how the real-world climate system responds to changing natural and anthropogenic external forcings, such as increasing greenhouse gases, land use and aerosols, as well as how the system also fluctuates due to its own chaotic internal variability. Similarly, individual climate model simulations also are a combination of the simulated forced response in the model and its simulated internal variability. Therefore, differences between observations and simulations may arise due to errors in the model’s external forcing and its simulated response to this forcing, but they may also arise due to an insufficient sampling of internal variability, an incorrect representation of the variability in the real world, or some combination of these factors.
To bridge these discrepancies, the authors developed a novel model evaluation framework exploiting the precise sampling of internal variability in large ensemble experiments. They evaluated ten climate models to determine whether real-world observations are well distributed within the climate states simulated by each model. This allowed them to attribute discrepancies between model simulations and observations to either biases in the simulated forced response or in the simulated internal variability, without the need to separate both signals in the observations.
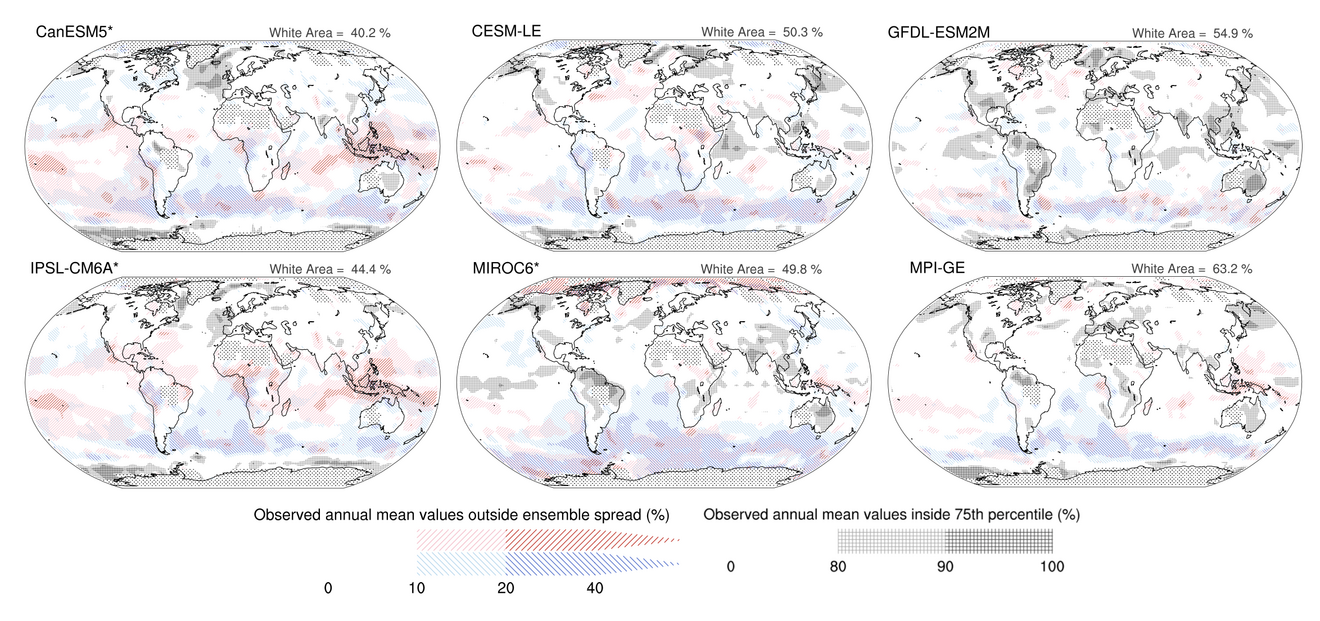
The authors found that, while some models fail to capture the long-term response to external forcing in global mean surface temperatures (GMST), none of them systematically under- or overestimate the range of internal variability in GMST. The largest discrepancies in GMST result from the overestimated forced warming in some models during recent decades. The Max Planck Institute for Meteorology Grand Ensemble (MPI-GE) is the ensemble with the most adequate representation of both the internal variability and forced response in observed GMST during the entire historical record, followed by the other large ensembles CESM-LE, GFDL-ESM2M, and IPSL-CM6A. On regional scales, the ensembles MPI-GE, GFDL-ESM2M, MIROC6, and CESM-LE capture the observed variability and forced response in historical surface temperatures most adequately, both in early as well as in recent periods. This indicates that, according to their evaluation metrics, MPI-GE, GFDL-ESM2M, and CESM-LE are the most adequate ensembles to investigate future projections of surface temperatures both globally averaged and globally at the grid-cell level.
The novel perspective of the authors on model evaluation offers new confidence in how comprehensive climate models capture the long-term trajectory of the climate system, as well as the range of possible fluctuations from this trajectory caused by internal variability in any given region and time period. Such an evaluation, both in terms of a model’s forced response and range of internal variability, allows the scientists to assess model performance more robustly than ever before. It is now possible to appropriately select which models are the best fit for different analysis in different regions of the globe, for studying current and past climate states, as well as future climate projections.
Original publication:
Suarez-Gutierrez, L., Milinski, S. & Maher, N. Exploiting large ensembles for a better yet simpler climate model evaluation. Climate Dynamics (2021). https://doi.org/10.1007/s00382-021-05821-w
Contact:
Dr. Laura Suarez-Gutierrez
Max Planck Institute for Meteorology
Email: laura.suarez@ mpimet.mpg.de
Dr. Sebastian Milinski
Now at National Center for Atmospheric Research (NCAR)
Email: sebastian.milinski@ mpimet.mpg.de
Dr. Nicola Maher
Now at University of Colorado, Boulder
Email: nicola.maher@ colorado.edu