Insights into the thermal structure of the tropical troposphere
Understanding the processes within the troposphere is vital for climate projections. The tropical troposphere is of special interest a) in itself because it covers a large part of the Earth where currently about 40% of its population live, and b) because tropical weather phenomena like El-Niño are known to affect weather and climate globally.
Theory suggests that greenhouse-gas-induced warming of the tropics should be strongest in the upper troposphere. However, for more than two decades a debate has been raging about apparent discrepancies in the representation of this climate change signal between climate models and observations. While the models in general show a clear amplification of the surface warming in the upper troposphere, this signal has been reported to be weaker or even absent in satellite observations. In recent years the gap has been reduced as retrievals of the temperature signal from satellites have improved, and as analysis methods have learned to take natural variability into account (e.g. Santer et al., 2017). However, uncertainty remains, a residue of still considerable gaps in our conceptual understanding of the processes that control the temperature structure of the tropical free troposphere. Over the past five years, these gaps have been a major focus of the research within the working group on Global Circulation and Climate, led by Dr. Hauke Schmidt, as a contribution to the Climate Physics research programme. Here we summarize results from a series of papers led by Jiawei Bao and Paul Keil. Their results are not only beneficial in the context of the above-described controversies, but advance the field more broadly as the lapse rate of the tropical tropopause, the rate at which temperature changes with height in the atmosphere, influences many aspects of the global circulation, e.g. the strength of the Walker circulation and its evolution under greenhouse gas forcing (Sohn et al. 2016), the atmospheric moisture flux into the Arctic (Lee et al. 2019) as well as tropical cyclone intensity (Trabing et al. 2019). Moreover, tropical cirrus clouds, an important factor for the atmospheric energy balance, depend on the lapse rate, or in other words on the stability, of the upper troposphere (Zelinka and Hartmann, 2010). A possible change of this lapse rate could lead to either more or less clouds, which can dampen or amplify global warming (Bony et al., 2016).
Two elementary ideas dominate the understanding of the temperature structure of the tropical free
troposphere: a) large horizontal gradients of density, and therefore also of temperature, are not sustainable in the tropical troposphere, which is referred to as the Weak Temperature Gradient (WTG) approximation, and b) the lapse rate follows a moist adiabat.
An adiabat is the temperature profile an air parcel would follow as it rises and expands through the atmosphere (or sinks and contracts) without an external input of energy. An air parcel moving upwards expands, and the work it does in this expansion is balanced by a reduction of its enthalpy, as measured by a lowering of its temperature. If this cooling leads to saturation of the water vapor in the parcel and hence condensation, the energy (or more precisely enthalpy) gained from the condensation is available to contribute to this work, resulting in less of a temperature reduction. The resulting vertical temperature profile is a moist adiabat.
However, little is known about the limits of these paradigms and how they may affect the temperature evolution of the free tropical troposphere under global warming.
In the following sections, we first review these elementary ideas and then present what we have learned from studies with a hierarchy of models on their limitations. The final three sections discuss changes in the lapse rate under warming, its relevance for surface climate change, and horizontal patterns of future temperature changes.
Weak temperature gradients and moist adiabats – a short introduction into tropical tropospheric temperatures
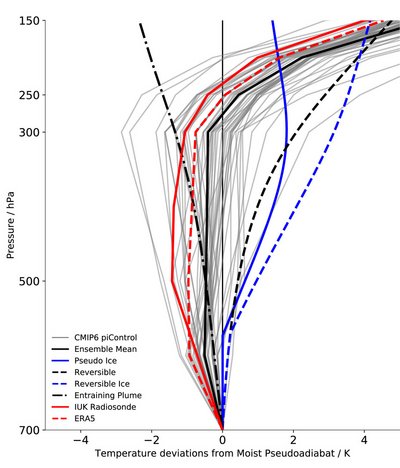
The first elementary idea on the temperature structure of the free tropical troposphere is based on the weakness of the Coriolis force in the tropics. This distinguishes the dynamics of this region from the extratropics where the large-scale circulation is largely geostrophic (pressure forces are balanced by the Coriolis force). Without such a substantial balancing force, as in the tropics, it is difficult to balance large changes in the mass field that arise by heating by changes in the circulation, and instead, gravity waves homogenize the mass and effectively reduce temperature gradients in the tropical free troposphere (Charney 1963; Bretherton and Smolarkiewicz 1989). The assumption of a horizontally relatively homogeneous temperature is the basis for the Weak Temperature Gradient approximation (WTG, Sobel and Bretherton 2000), which has been proven to be a useful approximation for analyzing tropical dynamics.
The second idea is that in regions of convective updrafts the vertical temperature profile, also called lapse rate, is defined by a moist adiabat. From the paradigm of horizontal homogeneity follows that the lapse rate should be, to first order, defined by a moist adiabat also in tropical regions away from convective updrafts. And indeed, moist adiabats describe the temperature decrease with altitude, which is larger than 100 K in the tropical troposphere, with an error of only a few Kelvin. However, it is known that this picture is very idealized. First, even under this theory, not temperature, but density should be homogenized horizontally. Because moist air is lighter than dry air, moist regions need to be colder than dry regions to have the same density. Another source of uncertainty comes from microphysical processes during the ascent, which leads to ambiguity in the definition of the moist adiabat. Different moist adiabats are shown in Fig. 1. A moist pseudoadiabat assumes that all condensate falls out immediately while a reversible adiabat assumes that all condensate is retained in the air parcel. This affects the heat capacity of the rising air parcel and amounts to a difference of several Kelvin in the upper troposphere. The freezing of condensate above the freezing level also offsets the need for cooling to balance the expansion work that is performed by a parcel as it ascends. And finally, in practice, a moist ascending air parcel would mix with surrounding drier air, a process called entrainment, which would increase cooling with altitude. Fig. 1 also shows how the temperature lapse rate of typical climate models, radiosondes and reanalysis data deviate from idealized moist adiabats. In the following sections, we will discuss, among other things, what may contribute to this spread.
Deviations from horizontally homogeneous temperature structure in storm-resolving models and reanalysis data
The causes for the spread of lapse rates across the models (Fig. 1) are difficult to determine. However, these models generally resolve the global atmosphere with a horizontal grid spacing of the order of 100 km or more. Towering clouds of tropical storms are the sign of convective updrafts responsible for the bulk of tropical vertical energy transport and, as described above, a key element for understanding the tropical temperature structure. Obviously, this process is occurring on smaller spatial scales than resolved by typical global models and its effects have to be parameterized. Keil et al. (2021) have shown that the spread of modeled lapse rates can be well reproduced by a single model in which a parameter characterizing the above-described entrainment process is varied. This shows that models with parameterized convection are of limited use for understanding the temperature structure of the tropical troposphere.
Global storm-resolving models, like ICON in its Sapphire configuration (Hohenegger et al., 2023), represent convection following the equations of motion and don’t require a parameterization of this process. Bao and Stevens (2021) have used ICON in a configuration with 2.5 km horizontal grid spacing to provide new insights into the dependence of the homogeneity of the tropical temperature on height. Fig. 2 shows that the tropical temperature distribution is much more homogeneous in the middle troposphere than in the upper troposphere. The maximum anomaly between the western and eastern Pacific is over 3.5 K at 300 hPa, but only about 1.5 K at 600 hPa. This homogenization difference is even more pronounced when density temperature, Tr, is considered which is more representative of actual density differences because it takes into account the relative lightness of moist air. The difference between altitudes indicates that the homogenization by gravity waves is less effective in the upper troposphere. Bao and Stevens (2021) speculate that this is due to the rareness of very energetic deep convection and a limitation of the influence of individual convective events on the neighborhood of the convection. Figure 2 shows also that generally, moister areas tend to be warmer, for reasons that cannot be explained purely thermodynamically. Two other studies described below have shed light on the causes of the inhomogeneities and the specific horizontal temperature patterns.
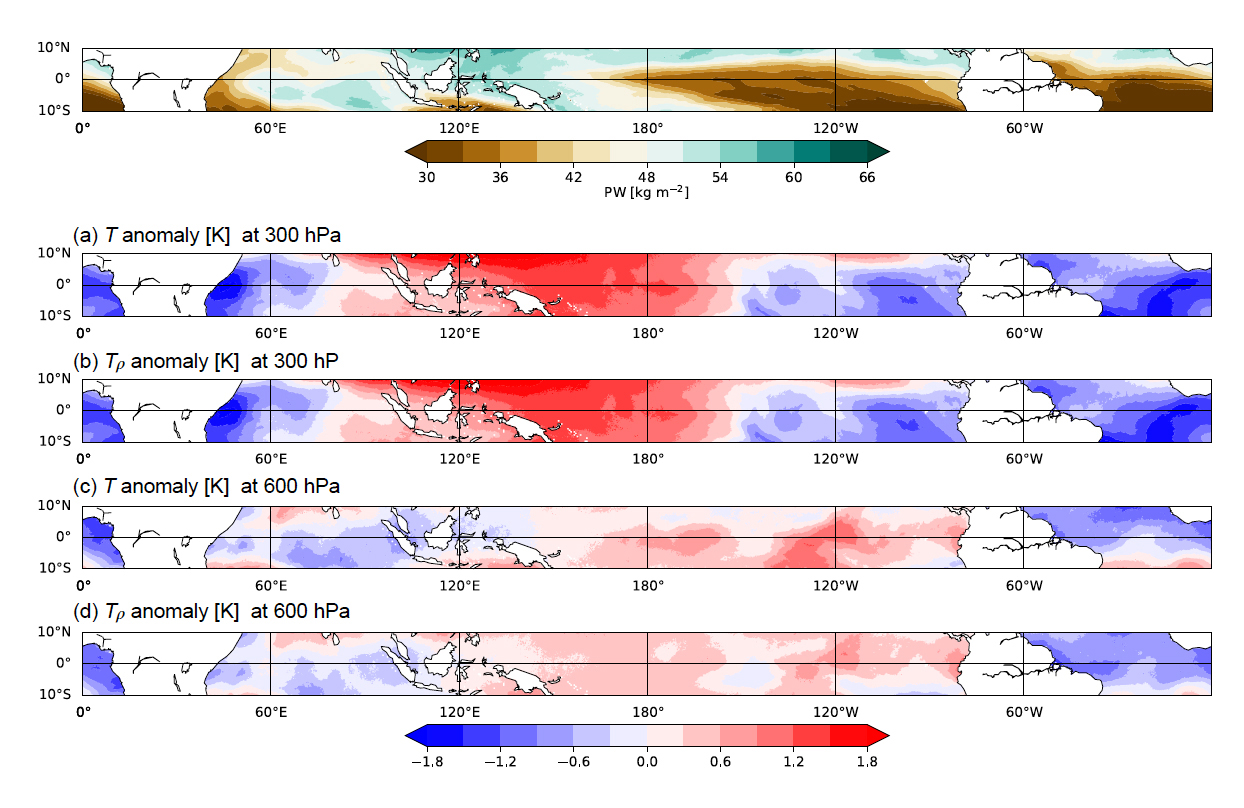
Beyond the relatively large-scale patterns visible in Fig. 2 the analysis of the ICON simulations reveals that the extremely moist regions, which are the regions of the strongest updrafts, are much warmer than the tropical mean. Already at 600 hPa, e.g. the 0.1 % moistest tropical columns have a more than 1K larger density temperature than the tropical mean. This shows that the tropical mean temperature is not defined by the few strongest updrafts but rather by the bulk of updrafts occurring also at somewhat smaller moisture content. The analysis of the vertical structure shows that in the moistest regions, the lapse rate is close to a reversible moist adiabat below the zero-degree altitude and close to a pseudoadiabat above.
The work from Bao and Stevens (2021) has demonstrated that weak horizontal temperature and density inhomogeneities can exist in kilometer-scale simulations of the tropics with ICON and that they are related to the distribution of moisture. However, it leaves open how big the inhomogeneities are in the real atmosphere and what sustains them. To answer these questions, Bao et al. (2022) analyzed reanalysis data. i.e. the state of a weather forecast model optimized to best match the observations, which provides arguably the best available representation of the real atmospheric state. In multi-year averages, temperatures at 500 hPa above the equator deviate nowhere by more than 1 K from the zonal mean. In extreme cases, monthly mean temperature differences between the west and central equatorial Pacific can reach up to 4 K. More importantly, the analysis of Bao et al. (2022) reveals a dynamical balance relevant for the tropics that sustains these temperature anomalies. The pressure gradients associated with the tropical temperature patterns are mainly balanced by the nonlinear terms in the momentum equation, in particular, provided by zonal wind advection, which is ignored in the geostrophic view.
The representation of the vertical structure influences its change under warming
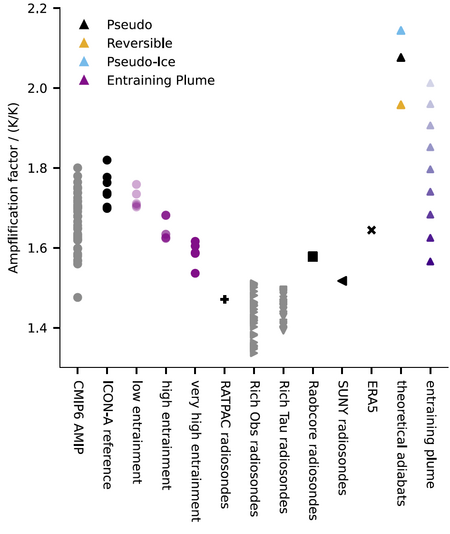
From the assumption that the tropical vertical temperature profile is close to a moist adiabat, it follows that the tropical troposphere should warm more strongly in its upper than in its lower part. The amplification of upper tropical tropospheric warming by models is generally stronger than observed. The reason for this is still debated. As said above, Keil et al. (2021) have analyzed the influence of parameter settings for entrainment of air into convecting plumes on the lapse rate simulated in classical atmospheric circulation models, and on the change of this lapse rate under warming. For comparison, they have not used satellite observations as done in many other studies, but they collected a variety of radiosonde data. Fig. 3 shows that in general also the analyses of radiosonde data, albeit having a large spread themselves, show weaker amplification than models. By varying the entrainment parameter in a single model (ICON) Keil et al. (2021) can produce a spread of amplification values that is similar to the spread of the multi-model CMIP6 ensemble. Simulations with stronger entrainment show weaker amplification because the amplification critically depends on the additional water vapor in a warmer atmosphere and entrainment reduces the available water vapor in the parcel. It has been suggested earlier that entrainment in models is often underestimated (Romps 2010). The results of Keil et al. (2021) suggest that this may contribute to the discrepancy between models and observations. Fig. 3 also shows that all of the idealized moist adiabats which ignore entrainment, show stronger amplification than both observed and simulated with climate models.
The representation of the tropical lapse rate is important for the simulation of the equilibrium climate sensitivity
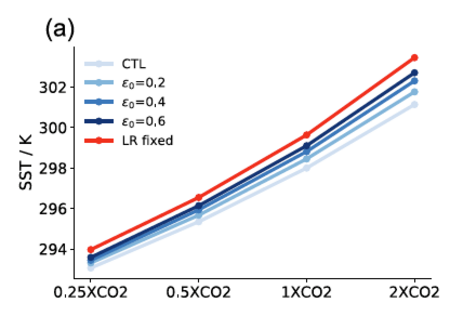
Above, it was discussed that entrainment contributes to the deviation of the tropical lapse rate from a moist pseudo adiabat and that different representations of entrainment in climate models also affect the change of the lapse rate with global warming. However, the lapse rate of the atmosphere also matters for the warming itself, because it influences how water vapor is distributed with temperature, and hence thermal emission to space. This then acts as a feedback on the surface warming. The sign and strength of this feedback are uncertain. To better quantify this feedback, and isolate the changes that determine it, Bao et al. (2021) have performed warming simulations with the one-dimensional radiative-convective equilibrium model “Konrad”, which has been developed at our institute as part of two PhD theses (Kluft et al., 2021, Dacie et al., 2021). Using such highly idealized tools in addition to more comprehensive three-dimensional models is often advantageous because the interpretation of their results is more straightforward and ideally the behavior of complex models, or observations, can be traced back to a few elementary processes. Fig. 4 shows the surface temperature simulated with Konrad for different atmospheric CO2 concentrations and with lapse rates determined for different assumptions on the strength of entrainment. Of course, temperature increases with CO2, but also with stronger entrainment. A larger entrainment causes a stronger temperature response to a doubling of CO2, i.e. a higher equilibrium climate sensitivity, suggesting that stronger entrainment acts as a positive feedback through its influence on how humidity is distributed with temperature in the upper troposphere. From the magnitude of this effect, derived from the idealized simulations, Bao et al. (2021) estimate that the spread of the lapse rate across CMIP models may contribute to a spread in clear-sky climate sensitivity of 0.2 to 0.3 K.
Future warming of the upper tropical troposphere will not be zonally homogeneous
The work from Bao and Stevens (2021) and Bao et al. (2022) has quantified existing temperature and density inhomogeneities in the tropical free troposphere and explained them. Depending on altitude they are related to the distribution of moisture and sustained by a dynamical balance different from geostrophic balance. It is also clear that the tropical mean upper tropospheric warming would be amplified compared to lower tropospheric warming, even if the magnitude of the amplification remains debated (Keil et al., 2021). How would the warming be distributed in the upper troposphere? Homogeneously? To answer this question, Keil et al. (2023) analyzed CMIP and storm-resolving ICON model simulations. Both show relatively inhomogeneous upper tropospheric warming. Under a homogeneous warming of sea surface temperatures by 4 K, e.g., the ICON model simulates warming at 300 hPa in the tropics that regionally differs by up to 2 K. Keil et al. (2023) then used the Matsuno-Webster-Gill theory (Matsuno 1966, Webster 1972, Gill, 1980), a very simplified representation of the equations describing the tropical circulation, to show that the warming pattern can be well reproduced when only the changes in condensation heating rates resulting from the precipitation changes under warming are used to force the model. Unfortunately, current models show a relatively large spread in simulating the distribution of tropical precipitation (Fiedler et al., 2020) and its future evolution. The upper tropospheric warming pattern under climate change can, hence, only be simulated more reliably if simulations of the precipitation change become more reliable. A reliable upper tropospheric warming pattern would also help to better constrain the amplification of upper tropospheric warming as measured by radiosondes. As tropical soundings are sparse and the warming pattern cannot be expected to be homogeneous, it is not easy to estimate how representative individual observations are for the tropical mean.
Summary and Outlook
Despite the progress described above, knowledge gaps on the thermal structure of the tropical troposphere, and with them our interest in this region of Earth’s atmosphere, remain. The discrepancy between observations and simulations concerning the amplification of surface warming in the upper tropical troposphere has been reduced in recent years, but the remaining differences are not negligible and merit an explanation. The work of Keil et al. (2021) emphasizes that tuning of classical atmospheric models, and in particular, their treatment of convective entrainment, does impact the simulated amplification strongly. A way forward is the use of models with a kilometer-scale horizontal resolution, like the Sapphire configuration of ICON, which avoids the need to parameterize convection and instead simulates this process, based on the laws of physics. This tool allows us to concentrate in the future on understanding the role of other processes, like cloud microphysics, and mesoscale circulations, which are also known to interact with the atmospheric thermal structure. Bao and Stevens (2021) showed that ICON’s Sapphire configuration is well suited to simulate and understand the deviations of tropical tropospheric temperature from horizontal homogeneity. The analysis of Bao et al. (2022) revealed a dynamical balance that sustains these inhomogeneities. Keil et al. (2023) showed that their future evolution clearly depends on the evolution of tropical precipitation patterns. The challenge to better understand tropical hydrology motivates a major field campaign, ORCESTRA, in the tropical Atlantic. ORCESTRA was initiated, and is co-led, by scientists from the department of Climate Physics, and will collect critical data in sparsely sampled regions to help better understand the processes that regulate the structure of the tropical troposphere
Publications
Bao, J., Dixit, V., & Sherwood, S. C. (2022). Zonal temperature gradients in the tropical free troposphere. Journal of Climate, 35, 4337–4348. https://doi.org/10.1175/JCLI-D-22-0145.1
Bao, J., & Stevens, B. (2021). The elements of the thermodynamic structure of the tropical atmosphere. Journal of the Meteorological Society of Japan, 99, 1483–1499. https://doi.org/10.2151/jmsj.2021-072
Bao, J., Stevens, B., Kluft, L., & Jiménez de la Cuesta, D. (2021). Changes in the tropical lapse rate due to entrainment and their impact on climate sensitivity. Geophysical Research Letters, 48, Article e2021GL094969. https://doi.org/10.1029/2021GL094969
Dacie, S., Kluft, L., Schmidt, H., Stevens, B., Buehler, S. A., Nowack, P. J., ... & Birner, T. (2019). A 1D RCE study of factors affecting the tropical tropopause layer and surface climate. Journal of Climate, 32(20), 6769-6782. https://doi.org/10.1175/JCLI-D-18-0778.1
Keil, P., Schmidt, H., Stevens, B., & Bao, J. (2021). Variations of tropical lapse rates in climate models and their implications for upper tropospheric warming. Journal of Climate, 34, 9747–9761. https://doi.org/10.1175/JCLI-D-21-0196.1
Keil, P., Schmidt, H., Stevens, B., Byrne, M. P., Segura, H., & Putrasahan, D. (2023). Tropical tropospheric warming pattern explained by shifts in convective heating in the Matsuno-Gill Model. Quarterly Journal of the Royal Meteorological Society, 149, 2678-2695. https://rmets.onlinelibrary.wiley.com/doi/10.1002/qj.4526
Keil, P. (2023). Tropical tropospheric temperatures, warming and involved mechanisms. PhD Thesis, Universität Hamburg, Hamburg. doi:10.17617/2.3509472.
Kluft, L., Dacie, S., Buehler, S. A., Schmidt, H., & Stevens, B. (2019). Re-examining the first climate models: Climate sensitivity of a modern radiative–convective equilibrium model. Journal of Climate, 32(23), 8111-8125. doi.org/10.1175/JCLI-D-18-0774.1
Other references
Bony, S., Stevens, B., Coppin, D., Becker, T., Reed, K. A., Voigt, A., & Medeiros, B. (2016). Thermodynamic control of anvil cloud amount. Proceedings of the National Academy of Sciences, 113(32), 8927-8932.
Bretherton, C. S., & Smolarkiewicz, P. K. (1989). Gravity waves, compensating subsidence and detrainment around cumulus clouds. Journal of Atmospheric Sciences, 46(6), 740-759.
Charney, J. G. (1963). A note on large-scale motions in the tropics. Journal of the Atmospheric Sciences, 20(6), 607-609.
Fiedler, S., Crueger, T., D’Agostino, R., Peters, K., Becker, T., Leutwyler, D., ... & Stevens, B. (2020). Simulated tropical precipitation assessed across three major phases of the Coupled Model Intercomparison Project (CMIP). Monthly Weather Review, 148(9), 3653-3680.
Gill, A. E. (1980). Some simple solutions for heat‐induced tropical circulation. Quarterly Journal of the Royal Meteorological Society, 106(449), 447-462.
Hohenegger, C., Korn, P., Linardakis, L., Redler, R., Schnur, R., Adamidis, P., ... & Stevens, B. (2023). ICON-Sapphire: simulating the components of the Earth system and their interactions at kilometer and subkilometer scales. Geoscientific Model Development, 16(2), 779-811.
Lee, S., Woods, C., & Caballero, R. (2019). Relation between Arctic moisture flux and tropical temperature biases in CMIP5 simulations and its fingerprint in RCP8. 5 projections. Geophysical Research Letters, 46(2), 1088-1096.
Matsuno, T. (1966) Quasi-Geostrophic Motions in the Equatorial Area. Journal of the Meteorological Society of Japan. Ser. II, 44, 25–43.
Romps, D. M. (2010). A direct measure of entrainment. Journal of the Atmospheric Sciences, 67(6), 1908-1927.
Santer, B. D., Solomon, S., Pallotta, G., Mears, C., Po-Chedley, S., Fu, Q., Wentz, F., Zou, C. Z., Painter, J., Cvijanovic, I. and Bonfils, C. (2017) Comparing tropospheric warming in climate models and satellite data. Journal of Climate, 30, 373–392.
Sobel, A. H., & Bretherton, C. S. (2000). Modeling tropical precipitation in a single column. Journal of climate, 13(24), 4378-4392.
Sohn, B. J., Lee, S., Chung, E. S. and Song, H. J. (2016) The role of the dry static stability for the recent change in the Pacific Walker circulation. Journal of Climate, 29, 2765–2779.
Trabing, B. C., Bell, M. M., & Brown, B. R. (2019). Impacts of radiation and upper-tropospheric temperatures on tropical cyclone structure and intensity. Journal of the Atmospheric Sciences, 76(1), 135-153.
Webster, P. J. (1972) Response of the tropical atmosphere to local, steady forcing. Monthly Weather Review, 100, 518–541.
Zelinka, M. D., & Hartmann, D. L. (2010). Why is longwave cloud feedback positive?. Journal of Geophysical Research: Atmospheres, 115(D16).
Contact
Dr. Hauke Schmidt
Max Planck Institute for Meteorology
hauke.schmidt@ mpimet.mpg.de
Prof. Dr. Bjorn Stevens
Max Planck Institute for Meteorology
bjorn.stevens@ mpimet.mpg.de
Dr. Jiawei Bao
now at: Institute of Science and Technology Austria (ISTA)
jiawei.bao@ ist.ac.at
Dr. Paul Keil
now at: Deutsches Klimarechenzentrum (DKRZ)
keil@ dkrz.de